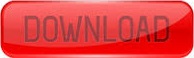

The camera installed on the line following robot aims to detect image-based lines and to navigate the robot to follow the path.

Robotic vision is a robot that can obtain information through image processing by the camera. However, it is irrelevant, along with the development of autonomous vehicles and robotic vision. The most widely used sensors for the robots are photoelectric sensors. Line following robot is one of the popular robots commonly used for educational purposes. Experimental findings from simulations and real-world settings showed the efficiency of using synthetic datasets in mobile manipulation task. The proposed system has been experimentally verified and discussed using the Husky mobile base and 6DOF UR5 manipulator. Second, the execution step takes the estimated pose to generate continuous translational and orientational joint velocities. First, a perception network trains on synthetic datasets and then efficiently generalizes to real-life environment without postrefinements. The developed system consists of two main steps. The proposed system integrates (1) deep-ConvNet training using only synthetic single images, (2) 6DOF object pose estimation as sensing feedback, and (3) autonomous long-range mobile manipulation control. Then, it is integrated into a 3D visual servoing to achieve a long-range mobile manipulation task using a single camera setup. In this paper, a deep-ConvNet algorithm is developed for object pose estimation. Incorporating the estimated pose into an autonomous manipulation control scheme is another challenge. However, visual pose estimation of target object in 3D space is one of the critical challenges for robot-object interaction.

The goal is to accomplish autonomous manipulation tasks without human interventions. The fourth industrial revolution (industry 4.0) demands high-autonomy and intelligence robotic manipulators.
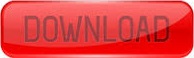